Registration for this module is now closed.
The purpose of this short course is to provide an overview of the analysis of longitudinal data in randomized controlled trials when outcomes are subject to irregular assessment times. Irregular assessment times are common when working with longitudinally collected outcomes; they may occur when patients do not return at precisely the protocol specified times due to schedule conflicts or sickness, or when patients visit their care provider between scheduled visits due to medical need. Just as missing data can lead to biased inferences about treatment effectiveness or prognosis over time, so too can irregular assessment times.
We will review the key steps in handling irregular assessment times. This begins with quantifying the extent of irregularity, then documenting the hypothesized relationships between outcomes, covariates, and assessment times. Given the hypothesized relationships, an analytic approach can be taken: we will review inverse-intensity weighted GEEs and joint models (both fully and semi-parametric). Sensitivity of inferences to untestable assumptions around the relationships between outcomes and assessment times should be examined, and we will describe how to examine sensitivity of inferences to the possibility of assessment at any given time t being dependent on the outcome at time t.
We shall assume that participants have MSc-level knowledge of statistics/biostatistics, a working knowledge of R, and are familiar with analysis of longitudinal data when observation times are regular, i.e. with GEEs and mixed models. We shall also assume familiarity with survival analysis and recurrent events. Since the course will involve hands-on analysis, participants are asked to have access to a computer with R packages IrregLong and SensIAT installed.
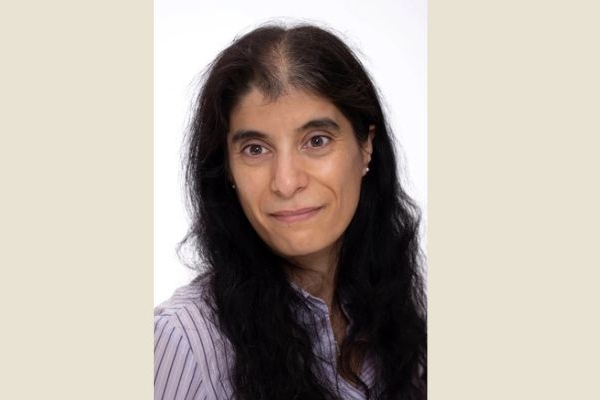
Eleanor Pullenayegum
Senior Scientist, Child Health Evaluative Sciences
The Hospital for Sick Children
Associate Professor, Institute of Health Policy, Management and Evaluation
Dalla Lana School of Public Health, University of Toronto
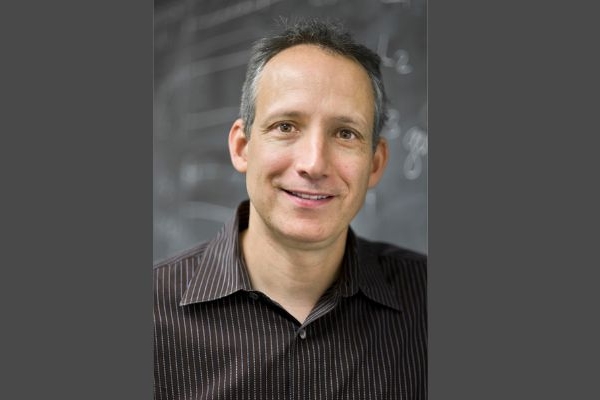
Daniel Scharfstein
Chief, Division of Biostatistics
Professor, Department of Population Health Sciences
University of Utah School of Medicine