Expanded content for 2025
In randomized clinical trials with baseline variables that are correlated with the outcome, there is potential to improve precision and reduce the required sample size by appropriately adjusting for these variables in the statistical analysis (called covariate adjustment). The resulting sample size reductions can lead to substantial cost savings, and also can lead to more ethical trials since they avoid exposing more participants than necessary to experimental treatments. Despite regulators such as the U.S. Food and Drug Administration and the European Medicines Agency recommending covariate adjustment, it remains underutilized leading to inefficient trials in many disease areas. This is especially true for trials with binary, ordinal, and time-to-event outcomes, which are quite common.
This module provides a comprehensive overview of covariate adjustment—explaining what it is, how it works, when it is beneficial, and how to implement it in a preplanned, model-robust manner across various scenarios. Using real-world trial data from multiple disease areas, we demonstrate its impact and practical application.
Hands-on applications will involve:
- Analysis of binary outcomes
- Analysis of time-to-event outcomes, especially when the proportional hazards assumption is violated
- Assessing precision gains through covariate-adjusted analyses
- Planning and implementing group sequential designs (GSD)
- Conducting and reporting GSD analyses with and without adjustment
- Planning information monitoring using both an interactive app and R command line
- Practical strategies for monitoring information and performing adjusted/unadjusted analyses
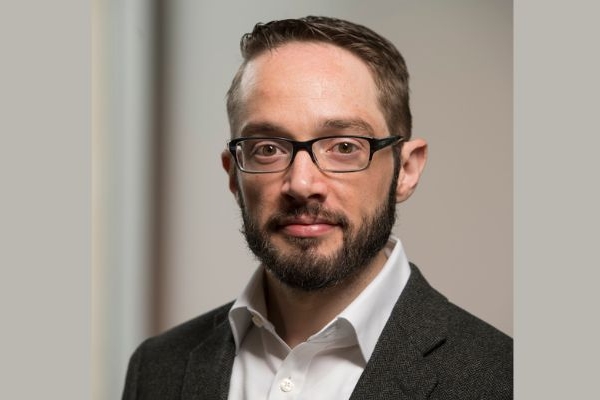
Joshua Betz
Associate Scientist, Department of Biostatistics
Johns Hopkins Bloomberg School of Public Health
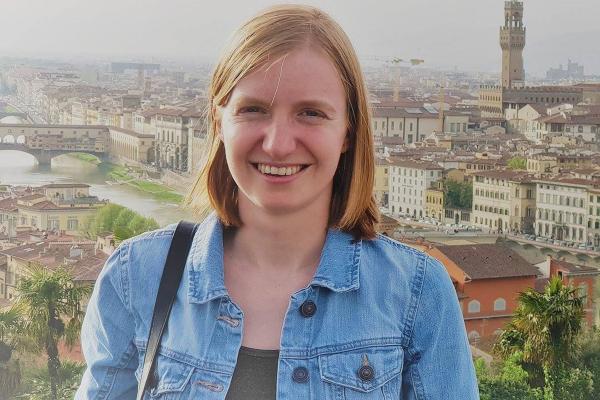
Kelly van Lancker
Research Scientist, Department of Applied Mathematics, Computer Science and Statistics
Ghent University